I said before that no one has yet patented a Meaning Machine. While that's true in the abstract, I want to talk about the two most common ways to hack around the problem. First is labor and second is scale.
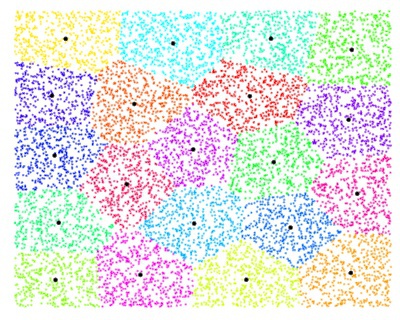
Meaning is the "hard problem" of computation, at least today. How do we know that data means something as opposed to something else? What's the difference between noise and signal? Is artificial intelligence (AI) able to discern meaning? And, perhaps more esoterically if not also pedantically, is meaning an analog technology or a digital technology? (For the final question, I take meaning formally as an analog technology, in that meaning entails a kind of Gestalt synthesis of complex arrangements of terms; yet practically speaking meaning is always the result of an interaction between the analog and the digital, and thus cannot be reduced to one or the other.)
Today there are two basic solutions to the "hard problem," the problem of meaning. The first solution is to outsource the problem to humans, effectively to make humans shoulder the burden of the Meaning Machine. To the extent that significance is measurable, it's because a human put it there and marked it as measurable. In other words, if you find meaning, it's the result of human labor.
Call it the Labor Theory of Meaning. And I mean labor very loosely here: any discernible action whatsoever is "labor" in the information economy, from emailing and tweeting, to click trails on websites, to larger things like authoring texts and tagging images and videos. A Mechanical Turk worker is a laborer in this sense, but so is anyone who has ever touched a computer or smart phone (as well as many who haven't). In the past, labor was characterized by its difficulty. Labor is still difficult today. Although even more difficult is not to perform labor, because it's very difficult not to be discernible.
(And "any discernible action whatsoever" really does mean any discernible action whatsoever. If one can discern a cell dividing or an electron leaving an atom, that division and that ionization qualify as "labor" under the current regime -- which is one explanation for why and how digital machines have become coterminous with the lowest kinds of discernibility we yet know, namely genetic codes and atomic states. Alternately, at conditions of indiscernibility -- entropy, randomness, contingency -- digitality ceases.)
The second solution to the problem of meaning we might call the Scale Theory of Meaning. At the Googleplex they say things like "quantity generates quality." If you want qualitative significance, they say, well then simply scale up! Raise the quantity to a few million data points, use a clustering algorithm like k-means, and qualities will naturally emerge. In other words Hume's problem (that causality is merely constant conjunction) is solved by a specific technology: scaling up. Don't forget that it has to be "big" data for them; little data is useless because it's low scale and therefore meaningless. Hence one of the basic dialectical contradictions of info capitalism: meaning is "up here" as the result of an analog event (integrating, synthesizing, organizing), even as the digital atoms remain "down there" (bits, keystrokes, sensor inputs). Both are necessary for the analog-digital dance to work properly. The Scale Theory of Meaning has been successfully implemented by Google, Amazon, and the last decade or two of info capitalism since the advent of Web 2.0.
Is there something wrong with the Scale Theory? No and yes. First it's important to acknowledge that scale is a technology, and an effective one at that. Scale is not simply a modifier for other tech, as with the Large Hadron Collider or Big Pharma. No, here scale is the tech. So the analog is an operation of synthesis. But it's also the operation of scaling-up. (Digitality, by contrast, entails down-scale operations like analysis and differentiation.)
What's wrong with the Scale Theory is that it's built on the backs of the Labor Theory. Scale hasn't solved the meaning problem, it has just outsourced the problem to millions of little agents. People still fabricate meaning; the machines simply glean it at scale. Remove the humans and you lose the meaningfulness. No humans, no signification. So the Scale Theory works, but only because it cheats. Scale obfuscates the true source of meaning, and sells the sleight of hand as "emergence."
To be sure, I'm not trying to evangelize humans or defend anthropocentrism here. Certainly we could expand this to mean "anything with an intentional sensory apparatus," which would include animals and plants and other kinds of nonhuman agents. If "human" gets used as a kind of convenient shorthand, it's only because I'm interested in the generation of coherent structure. But any structure of coherence will do.
If you run a clustering algorithm on a data source and find something meaningful in the data, that's only because some coherent structure put it there in the first place -- a person, an animal, a law of nature. To confirm this, run the same clustering algorithm on random data points; you'll still get perfectly valid outcomes just like before, except the outcomes will be meaningless.
In other words, measurement and meaning are two different things. A number alone can not tell you what it means. Why? Because meaning has been systematically excluded from the science of arithmetic since the ancients. To understand meaning you have to switch from a mechanical frame to a metaphysical frame, a frame that involves discrete numbers but is much larger than them. (Scientists and philosophers alike hate this about meaning; yet I suspect they actually just hate metaphysics and are taking out their frustrations on signification.) In short, scale/clustering won't confirm for you the validity of its own (valid) output.
This is true for companies like Google who rely on big data, but it's also true for AI. Remove the training data and the AI is effectively dead. Or, at best, it reverts back to the old-fashioned "symbolic AI" era of the 1960s -- and we know how that turned out. Today's AI is an extractive technology, in other words, and without meaning injected at the atomic level there's nothing to extract.
(People will object: yes but AlphaGo succeeded with no empirical training data! Perhaps, although I would define "no training data" a bit more rigorously. In fact, scientists furnished AlphaGo with a rich spectrum of concepts and relations from the history of human thought. There's no better training data than Leibniz's monad, the grid structure, and the Philosophical Decision, not to mention the rules for Go. But if AlphaGo can still win without being furnished with the foundations of digital philosophy, I'll eat my shoe.)
These kinds of humanist objections are already well known. Silicon Valley doesn't particularly care about the meaning problem. They don't much care about the labor problem either. They get their labor for free. And so they get their meaning for free as well. For Big Tech, correlation *is* causation, or at least it's measurably meaningful when parsed at scale. And that's all that matters. Scale is an analog technology, and an effective one at that, because it allows scientists -- and everyone else -- to merge multiple data points into one or more "emergent" qualities. The multiple becomes the one. Hence scaling-up is a version of the fundamental law of the analog, the Rule of One (2→1). And, for this, scale deserves a place in the Analog Hall of Fame.